[ad_1]
A brand new technique often known as YaRN (But One other RoPE for Transformers) has emerged, providing the potential to increase context capabilities in massive language fashions (LLMs) utilizing the RoPE method for positional coding. This method, as detailed in a latest article, offers the means to increase context as much as 64k and even 128k tokens. This innovation is especially notable because it addresses the rising demand for fashions that may accommodate substantial context, equivalent to prolonged texts or prolonged message histories.
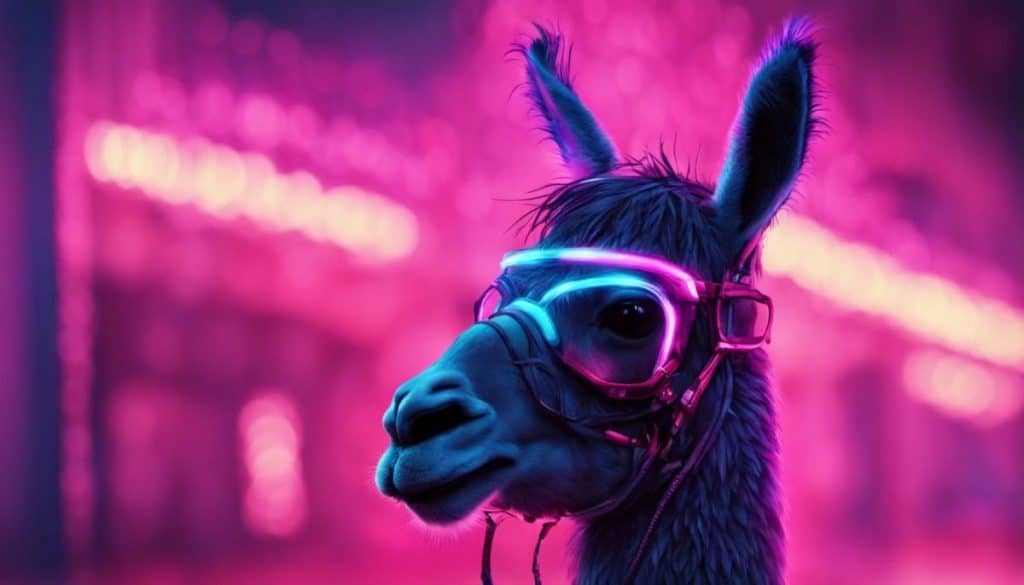
The RoPE technique entails rotating vectors in area at particular angles based mostly on their positions, and is especially utilized in fashions like LLaMa-2. The YaRN technique differs from earlier modifications, although, by including a brand-new element: a temperature parameter that’s essential in affecting how rapidly individuals listen after the softmax operation. This integration of temperature management is important as a result of it retains the eye mechanisms’ authentic construction and prevents the necessity for important modifications to the prevailing codebase.
An intriguing facet of YaRN’s implementation is its adaptability with current fashions hosted on platforms like Hugging Face. By harnessing the ability of those available fashions, researchers and practitioners can experiment with and discover the YaRN technique with relative ease.
It’s value noting that YaRN, like different novel methods, requires retraining on information containing prolonged contexts, albeit in a modest amount—roughly 0.1% of the pretraining information. The first consideration transferring ahead pertains to the computational assets crucial for effectively inferring with these expanded-context fashions, a side that may play a pivotal function within the sensible implementation of this revolutionary method.
YaRN opens the door to extra in depth contextual understanding, providing purposes that span varied domains, from literature evaluation to conversational AI. Because the AI group continues to discover strategies for enhancing mannequin capabilities, YaRN’s nuanced method to extending context holds the potential to supply beneficial insights and improved efficiency in varied pure language processing duties.In July, Meta has launched LLaMa-2-Chat fashions, a game-changing open-source language mannequin with 70 billion parameters, similar to and outperforming GPT-3.5 on sure benchmarks. The mannequin is commercially pleasant, pretrained on 2T tokens, and has sturdy MMLU scores. It’s the first mannequin of its measurement fine-tuned utilizing RLHF, making it fully free for business use. LLaMa-2-Chat showcases distinctive efficiency on mathematical issues and is offered in varied sizes.
Learn extra about AI:
[ad_2]
Source link