[ad_1]
Using a text-to-image diffusion mannequin, TokenFlow presents customers the chance to edit supply movies based mostly on particular textual content prompts. The end result? A refined video output that not solely aligns with the enter textual content immediate but additionally retains the unique video’s spatial configuration and movement dynamics. This achievement is grounded in TokenFlow’s principal statement: to keep up consistency within the edited video, it’s crucial to implement consistency inside the diffusion function house.
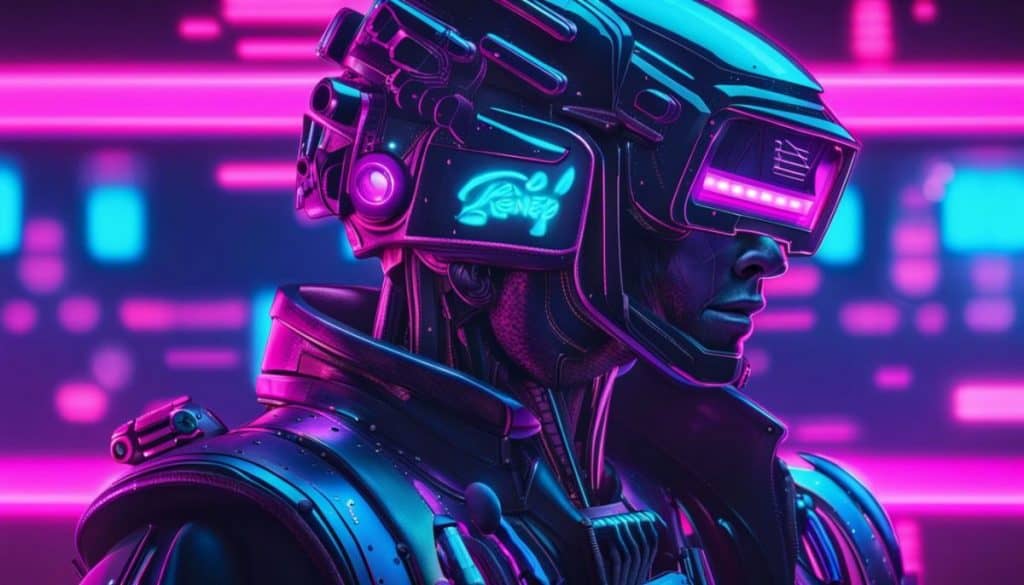
The strategy TokenFlow employs is each distinctive and environment friendly. As a substitute of counting on in depth coaching or changes, the framework leverages diffusion options derived from inter-frame correspondences inherent within the mannequin. This functionality permits TokenFlow to align seamlessly with pre-existing text-to-image enhancing strategies.
A deeper dive into TokenFlow’s methodology reveals its adeptness at sustaining temporal consistency. The framework observes {that a} video’s temporal consistency is intrinsically linked to its function illustration’s temporal consistency. Conventional strategies, when enhancing movies body by body, can usually disrupt this pure function consistency. TokenFlow, nevertheless, ensures that this consistency stays unaffected.
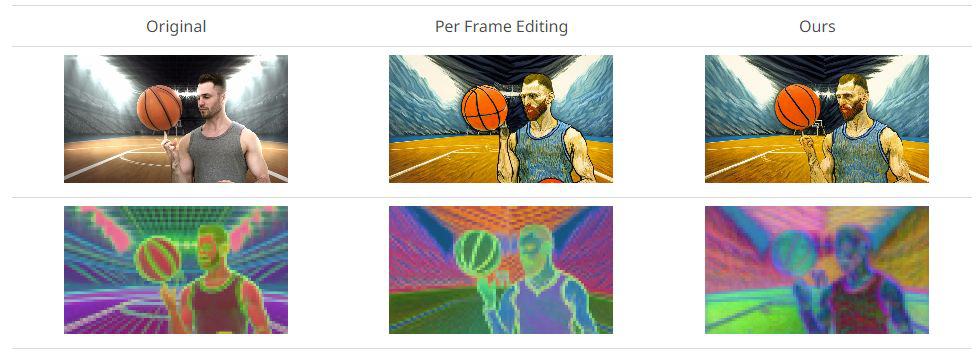
On the coronary heart of this course of is TokenFlow’s methodology of attaining a temporally-consistent edit. It does so by emphasizing uniformity inside the inner diffusion options throughout completely different frames in the course of the enhancing development. That is facilitated by the propagation of a particular set of edited options throughout frames, utilizing connections between the unique video options.
The method unfolds as follows:
For an enter video, every body is inverted to extract its tokens, primarily the output options from self-attention modules.Inter-frame function correspondences are then derived utilizing a nearest-neighbor search.Throughout denoising, keyframes from the video endure joint enhancing through an extended-attention block, resulting in the creation of the edited tokens.These edited tokens are then disseminated throughout the video, in keeping with the pre-established correspondences of the unique video options.
It’s noteworthy that TokenFlow’s method comes at a time when the generative AI sector is witnessing a shift in direction of video. The framework, with its concentrate on preserving the spatial and movement facets of enter movies whereas making certain constant enhancing, units a brand new normal. Furthermore, by eliminating the necessity for coaching or fine-tuning, TokenFlow proves its adaptability and potential to work in concord with different text-to-image enhancing instruments. This functionality has been additional exemplified by TokenFlow’s superior enhancing outcomes on a various vary of real-world video content material.
Learn extra about AI:
[ad_2]
Source link