In a current examination of the potential capabilities of huge language fashions, researchers problem the notion of “rising talents” and make clear a extra predictable side of their performance. The article titled “Unveiling the Realities of Massive Language Fashions’ Emergent Talents” brings to consideration the misinterpretation of metrics that has led to the misunderstanding that these fashions spontaneously purchase superior expertise.
The idea of “rising talents” within the context of huge language fashions, such because the GPT collection, has fueled considerations relating to the potential for these fashions to develop unexpected capabilities akin to human consciousness. This paper asserts that these assumptions have been primarily based on a flawed understanding of the fashions’ precise habits and capabilities.
The generally noticed phenomenon, the place bigger fashions seemingly purchase newfound talents equivalent to summary reasoning, problem-solving, and even humour, has been coined the “rising talents of Massive Language Fashions.” The authors of the article contend that these talents aren’t as spontaneous as they seem, however fairly a results of deceptive analysis metrics.
For instance their level, the researchers think about the duty of “guess the riddle,” an issue the place the language mannequin is required to grasp a pure language riddle and reply with the proper reply in pure language. Historically, the standard of responses has been evaluated utilizing a binary metric: a response is assigned a rating of 1 if it precisely matches the proper reply, and a rating of 0 in any other case.
The crux of the matter lies within the metric’s sensitivity to the complexity of the duty and the variety of mannequin parameters. The researchers reveal that this binary metric results in a misleading notion of “rising talents.” Smaller fashions typically exhibit negligible accuracy (eps) on this metric, whereas bigger fashions, significantly these with a excessive parameter rely, seem to realize outstanding accuracy ranges (acc > 0.5).
The article contends that this obvious shift in capability shouldn’t be indicative of fashions spontaneously buying complicated expertise. As an alternative, the fashions’ capability to know and generate extra nuanced responses stems from a extra meticulous analysis of their outputs. By specializing in probabilistic matching and semantic coherence fairly than precise string matches, the researchers present that the fashions’ development in efficiency follows a extra logical trajectory, no matter their dimension.
Investigating Mannequin Efficiency Evolution with Altering Parameters
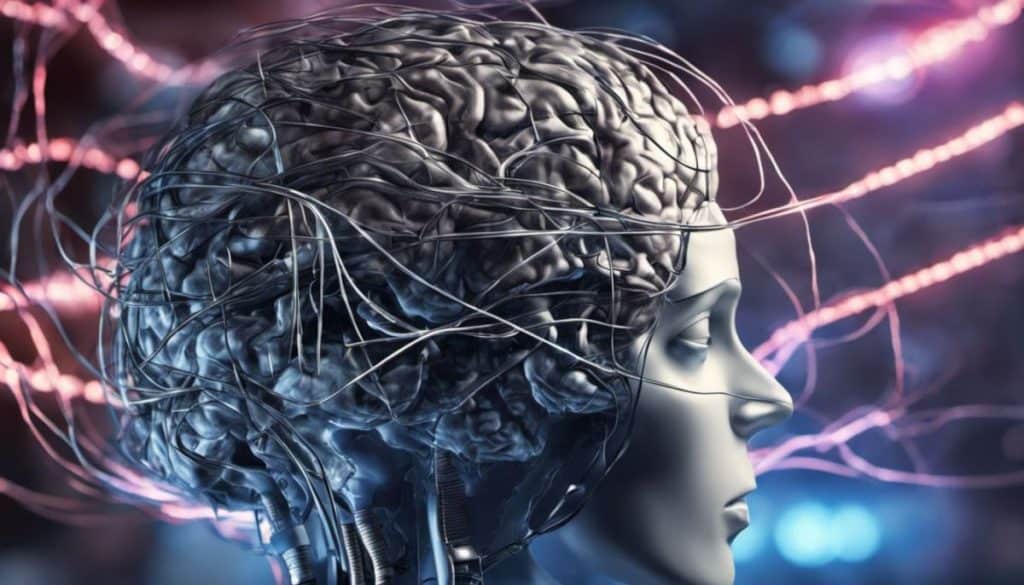
In an analytical investigation, researchers uncover the refined mechanics behind the perceived “rising talents” of huge language fashions. The examine questions the affect of superdiscrete metrics in evaluating mannequin efficiency and elucidates a extra predictive understanding of their capabilities as mannequin parameters increase.
The prevailing notion of “rising talents” in expansive language fashions has captivated discussions and raised considerations about potential breakthroughs. This examine seeks to disentangle the mechanics underlying this phenomenon and decipher whether or not these fashions certainly exhibit sudden, unprecedented capabilities or if these perceived developments may be attributed to a distinct trigger.
On the coronary heart of the examine lies a meticulous analysis of the metrics employed to gauge mannequin efficiency. The researchers contend that the usage of superdiscrete metrics, significantly the standard binary metric that determines precise string matches, would possibly distort the interpretation of huge language mannequin talents. The examine meticulously analyzes how the chance distribution of model-generated solutions evolves as mannequin parameters scale.
Opposite to the notion of “rising talents,” the examine reveals a extra systematic development. As the scale of the mannequin will increase, its capability to assign increased possibilities to acceptable solutions and decrease possibilities to incorrect ones improves. This displays a constant enhancement within the mannequin’s capability to resolve issues adeptly over a variety of sizes. In essence, the analysis means that the fashions’ studying course of follows a well-defined trajectory of enchancment fairly than a sudden leap.
The authors introduce a paradigm shift by proposing the substitute of discrete metrics with steady ones. This transformation affords a clearer image of efficiency evolution. Via their evaluation, the researchers confirm that roughly 92% of the Huge Bench issues exhibit a clean and predictable development in high quality as mannequin dimension expands. This discovering challenges the notion that bigger fashions expertise sudden breakthroughs and as a substitute highlights a extra gradual and anticipated development.
The examine extends its insights to validate its claims. It demonstrates that the identical “rising capability” impact may be artificially simulated utilizing standard autoencoders, suggesting that the selection of metrics considerably influences the perceived outcomes. This revelation broadens the scope of the examine’s implications, demonstrating its relevance past language fashions alone.
The researchers emphasize that their outcomes don’t definitively negate the potential for “rising talents” or consciousness in giant language fashions. Nonetheless, their findings do encourage researchers to strategy such claims with a nuanced perspective. Reasonably than unexpectedly extrapolating and forming excessive conclusions, the examine underscores the significance of meticulous investigation and complete evaluation.
Learn extra about AI: