[ad_1]
On the planet of AI’s vivid future, everybody solely talks concerning the gorgeous results of information evaluation and what knowledge groups can deliver to the desk usually. Have you ever seen these results in actuality: particular money flows because of the implementation of information evaluation tasks? The reply is probably going ambiguous. So Gartner mentioned the issue of evaluating the results of information groups at their main convention on knowledge and analytics this yr.
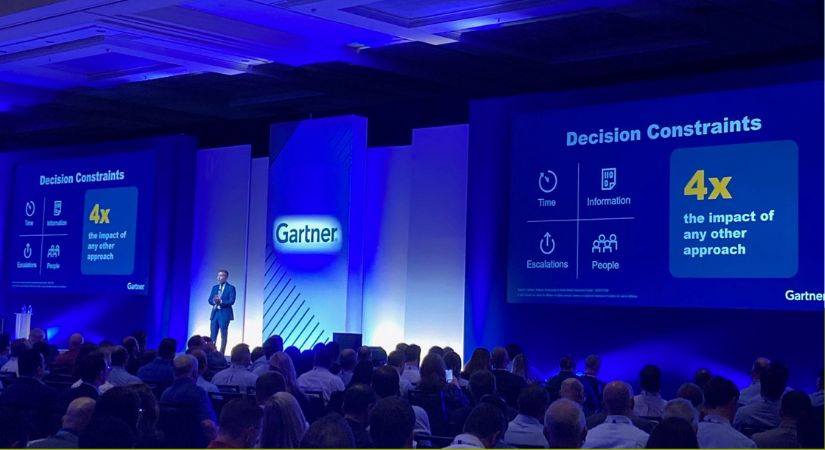
Based on Gartner’s examine, since 1975, there was a gentle decline within the proportion of firms that measure the particular monetary impression of information analytics tasks (income progress, price discount, productiveness progress, and danger discount). Already in 2020, greater than 90% of investments in knowledge (in opposition to 17% in 1975) had been justified by the so-called strategic targets: the creation of improvements, knowledge as an asset, and model worth.
After which you’ll be able to discuss so much about how and why we got here to this and what’s going to occur subsequent in opposition to the backdrop of the gathering clouds within the world macroeconomic setting.
Why has the development fashioned?
Justifying the impact of information evaluation by way of strategic targets is, in lots of instances, fairly regular. The event of the business lately has already develop into apparent to everybody, it appears: ChatGPT right here makes the final shot the final doubter. In the meanwhile of a breakthrough, no firm that wishes to outlive needs to stay hopelessly behind.
Justifying the impact with strategic targets is usually pressured when you don’t spend money on understanding what actual monetary results investments in knowledge can deliver and the way this may be measured. Many firms make investments large figures in tasks to enhance enterprise processes based mostly on knowledge, however on the similar time, they save on creating a strategy for evaluating the results of those tasks (AB testing, post-investment evaluation of information tasks, and so forth.). With every new mission, such firms get increasingly slowed down within the entice of uncertainty; for them, the chance of the ultimate chapter of all knowledge exercise is rising, or the info staff is overinflated with out understanding the success of their actions.
On the similar time, in observe, the introduction of such methodologies has all the time resulted within the biggest results on all knowledge tasks.
What is going to occur subsequent?
The darkish aspect is the rising vulnerability of information groups in a tough macroeconomic state of affairs on world markets. If 90% of the results of some kinds of groups can’t be “touched” as a result of they’re someplace within the vivid future, when the financial disaster intensifies, it is going to be these groups that would be the first to be hit. Sadly, the start of this development was largely confirmed by 2022, and various large-scale layoffs in massive firms.
The brilliant aspect is the elevated curiosity in actual monetary impression assessments. Towards the background of all the above, we count on that in 2024–2025 there will likely be a development reversal, and extra investments will likely be justified by an actual monetary impact.
And this can imply a rise in curiosity in strategies like Dependable ML: tips on how to set up the work of information groups in order that the impact of their actions is measurable and financially optimistic. To do that, it is advisable take into consideration ML system design (so as to not get into clearly unprofitable or unrealizable tasks), causal inference (so as to not fall into the entice of false patterns), and AB testing (with a purpose to appropriately perceive whether or not your prototype will deliver cash when scaling).
Learn extra associated information:
[ad_2]
Source link