[ad_1]
Chinese language researchers have unveiled a brand new LLM, the FLM-101B, a decoder-only LLM boasting a outstanding 101 billion parameters. This growth gives a cheap different for each analysis and sensible functions.
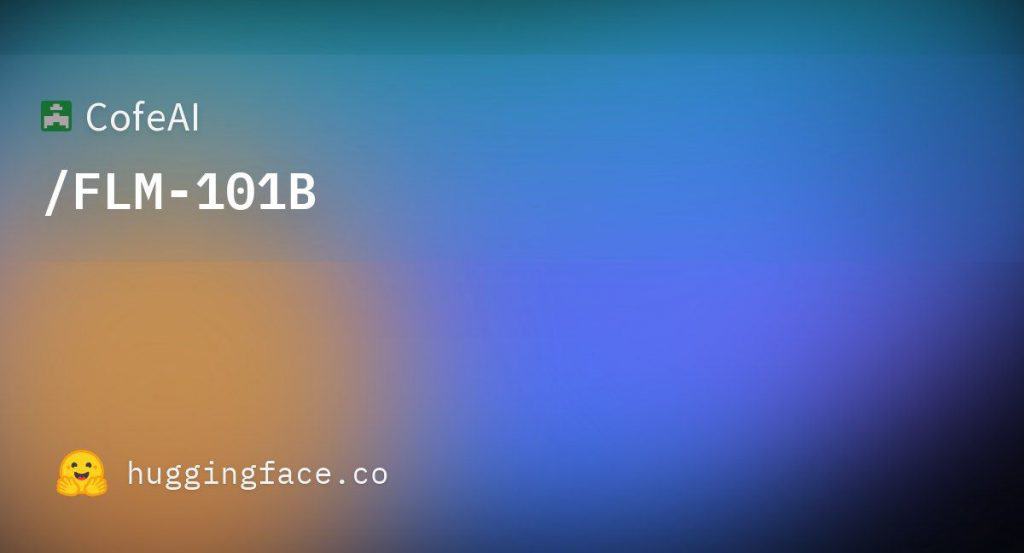
What makes FLM-101B stand out is its distinctive efficiency achieved on a comparatively modest funds. Whereas it’s well-known that coaching LLMs from scratch can require astronomical investments, the creators of FLM-101B have proven that it’s attainable to coach a mannequin with 101 billion parameters utilizing only a $100K funds.
The experimental outcomes are nothing in need of spectacular. FLM-101B has demonstrated efficiency ranges similar to established and resource-intensive fashions like GPT-3 and GLM-130B. This comparability highlights the large potential of this cost-effective mannequin, notably on IQ benchmarks with advanced contexts not current within the coaching knowledge.
In a transfer that underlines their dedication to advancing AI analysis and growth, the creators of FLM-101B have made this mannequin open-source. Researchers and builders worldwide can now entry and leverage this 101B-scale LLM for varied functions, spanning each the Chinese language and English languages.
The FLM-101B mannequin employs a singular coaching method. It quickly accumulates information from a smaller 16-billion-parameter mannequin within the preliminary phases of coaching and progressively scales as much as 101 billion parameters. This incremental method considerably reduces coaching prices, making it financially possible for a broader vary of tasks.
One standout function of FLM-101B is its assist for environment friendly window measurement growth throughout inference. That is achieved by using xPos rotary place embedding, permitting the mannequin to deal with a broader context, enhancing its adaptability and value.
FLM-101B was educated on a cluster of 24 DGX-A800 GPU servers in lower than 26 days. This spectacular feat underscores the mannequin’s scalability and environment friendly useful resource utilization. The mannequin’s coaching codebase, tailored from Megatron-LM, will quickly be out there as open-source, offering useful insights for the AI neighborhood.
The creators of FLM-101B acknowledge potential limitations, together with the mannequin’s publicity to unsafe examples within the coaching corpus because of the open nature of the dataset. This caveat serves as a reminder of the significance of accountable AI utilization and content material moderation.
Whereas FLM-101B has achieved outstanding outcomes, the creators acknowledge areas for enchancment. The mannequin’s inference course of, whereas highly effective, will not be but absolutely optimized, resulting in larger useful resource utilization and lowered pace. Nonetheless, plans are underway to introduce Flash Consideration in inference, addressing this limitation.
Learn extra about AI:
[ad_2]
Source link